Head and neck
Online First
Identification of IGF2BP2 and long non-coding RNA TUG1 for the prognosis and tumour microenvironment in head and neck squamous cell carcinoma
Abstract
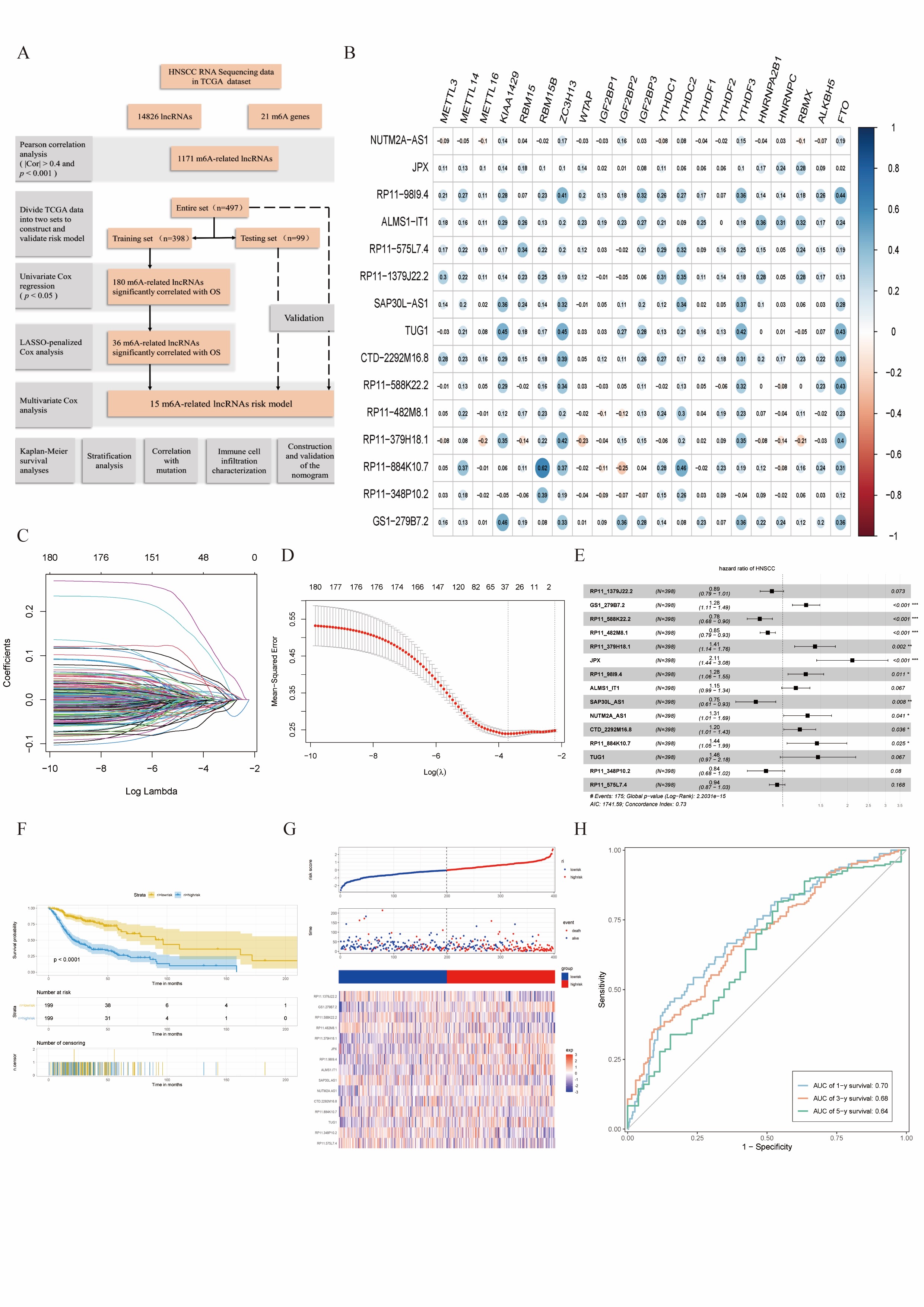
Objective. This study aimed to investigate the role of m6A-related long non-coding RNAs (lncRNAs) in the prognosis and tumour microenvironment of head and neck squamous cell carcinoma (HNSCC).
Methods. 497 samples from The Cancer Genome Atlas were analysed to identify m6A-related lncRNAs via correlation models. Tripartite regression models, Kaplan-Meier analysis and nomograms were then utilised to assess the prognostic significance of these lncRNAs. Tumour mutation burden and immune cell infiltration analyses were also performed. Moreover, m6A-related lncRNAs expression and relation with IGF2BP2 were confirmed by RT-qPCR.
Results. The risk model revealed that high-risk scores predicted poorer survival outcomes. The area under ROC curves for predicting 1-, 3-, 5-year survival in the training set were 0.70, 0.68, and 0.64, respectively. Seven key m6A-related lncRNAs showed associations with immune checkpoint molecules, especially CTLA4 and PD-1. Finally, we found that knockdown of TUG1 repressed the expression of IGF2BP2.
Conclusions. Our results suggest that the m6A-related lncRNA risk model has potential clinical utility in predicting prognosis and immunotherapeutic responses in patients with HNSCC. Identification of candidate compounds for immunotherapy further emphasises the model’s relevance in guiding treatment decisions for HNSCC.
Introduction
Head and neck squamous cell carcinoma (HNSCC), the most common head and neck tumour, develops from mucosal epithelium in the oral cavity, pharynx, and larynx. Due to high recurrence rates and resistance to chemotherapy, patients with HNSCC have poor survival rates and limited treatment options. Therefore, it is urgent to search for novel biomarkers to predict prognosis and identify targets for immunotherapeutic strategies.
Long non-coding RNAs (lncRNAs) predominantly associate with many cellular processes, and aberrantly expressed lncRNAs have been shown to regulate growth of HNSCC. For example, lncRNA HOX transcript antisense RNA has been reported to modulate the progression of HNSCC 1. LINC00355 is a promoter of HNSCC by hindering microRNA-195-mediated suppression of HOXA10 expression 2.
N6-methyladenosine (m6A) is a dominant and abundant transcriptome modification. m6A mediates various aspects of RNAs (such as RNA translation, processing, transport, and stability) by effector proteins (“writers”, “readers”, and “erasers”) 3. As has been shown previously, m6A modification of lncRNAs plays a critical role in cancer 4 and m6A modification is vital in the enrichment and regulation of lncRNAs 5. m6A modification regulates lncRNAs at specific DNA sites and induces the binding of RNA-binding proteins via providing the ”reader” with a binding site or regulating the structure of local RNA 6. Nevertheless, the specific role of m6A effectors in lncRNAs in HNSCC remains unclear.
The tumour microenvironment (TME) is emerging as a new immunotherapeutic strategy to monitor tumour growth, and many lncRNAs participate in intracellular signaling in the TME. Recently, accumulating studies have suggested that lncRNAs are critical regulators of tumour development and cancer-associated immune responses 7,8. However, little is known about the association between lncRNA expression patterns and TME in HNSCC.
Aberrantly expressed lncRNAs and the TME are tightly implicated in progression and prognosis of HNSCC. Therefore, it is useful to identify targets through exploring the mechanism of m6A-related lncRNAs in HNSCC progression. This work systematically assessed the effects of m6A-related lncRNAs on the prognosis and TME of HNSCC based on The Cancer Genome Atlas (TCGA) dataset (n = 497). Moreover, the m6A-related lncRNA risk model and the relationship of m6A-related lncRNAs with the TME were explored to forecast the overall survival (OS) of patients with HNSCC. Finally, a nomogram was established.
Materials and methods
Datasets information and selection of m6A-related lncRNAs
RNA sequence transcriptome data, clinical information, and mutation data of HNSCC patients were obtained from the TCGA database (https://portal.gdc.cancer.gov/). Clinical information contained sex, age, TNM classification, tumour stage, survival outcomes and OS. HNSCC patients with missing OS values or OS < 0.1 month were excluded to reduce statistical bias in our analysis. Ultimately, 497 patients were included and allocated (training set: testing set = 8:2). In detail, 133 patients were females and 364 patients were males; 244 were 60 years of age or younger, whereas 253 were older than 60 years. A total of 282 individuals were living and 215 had died. Regarding tumour stage, 94 were categorised as either stage I or II, 365 were classified as stage III or IV, while staging information for 68 patients was unknown. In terms of TNM classification, 156 were T1 or T2, 258 were T3 or T4, and while T category was unknown in 83 patients. Lymph node involvement was categorised as N0 or N1 in 221 patients, N2 or N3 in 226 patients, and unknown for 113 individuals. Additionally, the metastasis status was M0 for 163 patients and M1 for one patient, and for 333 patients was unknown. From the TCGA database, we extracted expression matrixes of 21 m6A-related genes. In all, 1171 m6A-related lncRNAs were identified via the criteria: (1) Pearson’s correlation analysis and |Pearson R| > 0.4, (2) p < 0.001.
Establishment and validation of the risk model
The entire TCGA set was randomly allocated into a training set and testing set at a ratio of 8:2. The training set was utilised for m6A-related lncRNA risk model construction, which was then validated using the entire set and testing set. According to HNSCC survival information in TCGA, the prognosis of 1171 m6A-related lncRNAs was screened (p < 0.05). Univariate Cox regression and LASSO-Cox regression were conducted, and 36 m6A-related lncRNAs were found to be distinctly related to the OS of patients. A 15-m6A-related lncRNA risk model was ultimately established after analysing the 36 m6A-related lncRNAs via multifactor Cox regression. The risk score was calculated with the formula reported previously 9. The groups were divided into low and high-risk based on the median risk score.
Exploration of the model in the immunotherapeutic treatment
The R package maftools was used to explore mutation data. The tumour-specific mutated genes were found and TME was evaluated.
Kaplan-Meier survival analysis
Kaplan-Meier survival analysis was employed to evaluate the diversity in the OS between the high-risk and low-risk groups. The R packages survMiner and survival were tools used in this process.
Independence of the m6A-related lncRNAs risk model
To test whether the risk model has prognostic pattern and variable was independent, the multivariate and univariate Cox regression analyses were conducted.
Establishing and proving a predictive nomogram
The nomogram showed predictive ability of the m6A-related lncRNA risk model, and the 1-, 3-, and 5-year OS were set up.
Clinical tissue sampling
Four laryngeal cancer tissue samples and four adjacent noncancerous tissue samples were collected from October 2021 to January 2022 from patients who underwent surgical resection at Second Xiangya Hospital with the approval of the Ethics Committee of Second Xiangya Hospital. Written informed consent was obtained from all patients enrolled, and the ethics acceptance ID was 2019EA.NO(R008). All tissue samples were preserved by snap freezing and kept at -80°C.
Cell culture and transfection
Human bronchial epithelial cell line 16HBE (SCC150) was procured from Sigma-Aldrich (St. Louis, MO, USA) and cultivated in Dulbecco’s Modified Minimum Essential Medium (Corning, Manassas, VA, USA) with 10% foetal bovine serum (FBS; Corning). The TU686 cell line was procured from Xiangya Cell Bank (Central South University, Changsha). Cells were cultivated in RPMI1640 media containing 10% heat-inactivated FBS with penicillin/streptomycin at 37°C in 5% CO2. The IGF2BP2 siRNA (siIGF2BP2 #1 and siIGF2BP2 #2) was produced by GenePharma (Shanghai, China). Lipofectamine 3000 Reagent (ThermoFisher Scientific, Waltham, MA, USA) was employed to perform all cell transfections.
2.9 qRT-PCR
Total RNA was isolated from target tissues or cells using Trizol reagent (Invitrogen, Waltham, MA, USA). The expression levels of target factors were evaluated with a SYBR Green qPCR assay (Takara, Dalian, China). For data processing, the 2-ΔΔCT method was used taking α-tubulin expression as an endogenous control.
Statistical analyses
Data from three independent experiments were expressed as the mean ± SD and processed using SPSS17.0 statistical software (IBM Corporation, Armonk, NY). Differences between two groups were compared using the Student’s paired test. Differences among more than two groups in the above assays were estimated using one-way ANOVA. A p value < 0.05 was considered significant.
Results
Selection of m6A-related lncRNAs
Combined with the Pearson’s correlation analysis, the m6A-related lncRNAs were derived from the TCGA database and associated with one of the 21 m6A genes (|Pearson R| > 0.4 and p < 0.001). After identifying 21 m6A genes and 14,826 lncRNAs, we obtained 1,171 m6A-related lncRNAs. The work flow is shown in the Cover figure (A).
Construction of the m6A-related lncRNAs risk model
In the TCGA training set, m6A-related prognostic lncRNAs were screened out from 1171 m6A-related lncRNAs (p < 0.05). A total of 180 m6A-related lncRNAs depicted correlation with the OS in patients with HNSCC. For the subsequent multivariate analysis, 36 m6A-related lncRNAs were selected by LASSO-penalised Cox analysis (Cover figure, C-D). Combined with 36 m6A-related prognostic lncRNAs, we constructed a risk model including 15 m6A-related lncRNAs to assess the prognostic risk of patients with HNSCC by multivariate Cox ratio hazard regression analysis. Cover figure B shows that the main m6A-related lncRNAs had positive correlation with 21 m6A genes. The Forest plot showed that RP11_1379J22.2, RP11_588K22.2, RP11_482M8.1, SAP30L_AS1, RP11_348P10.2 and RP11_575L7.4 were protective factors with HR (Hazard ratio) < 1, while GS1_279B7.2, RP11_379H18.1, JPX, RP11_98I9.4, ALMS1_IT1, NUTM2A_AS1, CTD_2292M16.8, RP11_884K10.7 and TUG1 were risk factors with HR > 1 (Cover figure, E). In the TCGA training set, patients were grouped according to median value of risk scores. HNSCC patients in high-risk subgroups harboured lower OS rates (Cover figure, F). The patients with high-risk score showed worse survival status distributions, and some m6A-related lncRNAs had different relative expression standards between intra-group (Cover figure, G). The ROC curves showed that m6A-related lncRNAs risk model had strong predictive ability in TCGA training set, as shown by the area under ROC values of 0.70, 0.68, 0.64 for 1-, 3-, and 5-year survival (Cover figure, H).
Validation of the m6A-related lncRNAs risk model
Figures 1A and B showed the relationship between m6A-related lncRNAs expressions and survival information in the testing set (Fig. 1A) and entire set (Fig. 1B). Risk scores for each patient in the testing set and entire set demonstrated this model had good prognostic capability. HNSCC patients with higher risk scores had worse OS in the testing set and entire set in accordance with the findings in the TCGA training set (Fig. 1C-D).
Prognostic analysis and stratification analysis of the m6A-related lncRNAs and risk model
The subgroups classified by clinical information of the low-risk group continued to be superior to that of the high-risk group in the TCGA entire set (Fig. 1E). Kaplan-Meier survival analyses confirmed that higher expression of RP11_1379J22.2, RP11_588K22.2, RP11_482M8.1, SAP30L_AS1, RP11_348P10.2 RP11_575L7.4, RP11-379H18.1 and RP11-884K10.7, and lower expression of NUTM2A-AS1, JPX, RP11-1379J22.2, SAP30L-AS1, RP11-482M8.1, RP11-348P10.2 and GS1-279B7.2 predicted better OS in the TCGA dataset (Fig. 1F).
Tumour mutation burden analysis and evaluation of immune cell infiltration characterisation
After examining the mutation data from the TCGA database via “Maftools” R package, the waterfall plot showed the mutation data for the top 20 genes in each sample (Fig. 2A). Moreover, mutations were grouped, and missense mutations and single nucleotide polymorphisms (SNP) and C > T occurred more often. Figure 2B revealed mutation types based on the number of changed bases in each sample and the top 10 mutated genes in HNSCC, and TP53 (67%) was much higher than the other mutations. The correlations of the TCGA entire set between the top 30 and 15 m6A-related lncRNAs are shown in Figure 2C, and the coexpression network among mutated genes, m6A-related lncRNAs and m6A was visualized using the Sankey diagram in Figure 2D.
As shown in Figure 3A, only 19 cell subtypes presented significant differences after evaluating 28 TME cell subtype infiltrations between normal and tumour samples. Only immature dendritic cells, plasmacytoid dendritic cells, effector memory CD4+ T cells, eosinophils and mast cells were abundant in normal tissues. We performed Spearman correlation analyses, and the main key molecules negatively correlated with TME infiltrating cells and 14 m6A-related lncRNAs exhibited a prominent negative correlation with neutrophil infiltration (Fig. 3B). Moreover, we noted the expression of 7 key m6A-related lncRNAs with immune checkpoint molecules, especially CTLA4 and PD-1 (Fig. 3C). Of these, RP11_588K22.2 was particularly associated with CTLA4 and PD-L2 (Fig. 3D).
Univariate Cox regression analysis and multivariate Cox regression analysis
The risk model of 15 m6A-related lncRNAs had independent prognostic factors for HNSCC, and could be a tool to assess clinical prognosis. The HRs of the risk score were 1.373 and 1.317, and 95% confidence interval (CI) were 1.295−1.456 and 1.234−1.405 in univariate Cox regression analysis (Fig. 3E) and multivariate Cox regression analysis (Fig. 3F), respectively.
The prognostic nomogram
We constructed the nomogram containing the risk grade and clinical factors which had predominant predictive ability (Fig. 3G). Correlation charts displayed that the observed versus predicted rates of 1-, 3-, and 5-year OS revealed ideal consistency (Fig. 3H).
Validation of lncRNAs expression and relation with IGF2BP2
Two m6A-related prognostic lncRNAs (TUG1 and JPX) and m6A effector protein (IGF2BP2) expression levels were detected in laryngeal cancer samples using RT-qPCR assay. Our results showed that TUG1, JPX and IGF2BP2 were upregulated in laryngeal cancer samples compared with normal tissues, which showed the same expression trend in TCGA database including 43 paired samples (Fig. 4A). According to the RNA sequences of TUG1 and JPX, we predicted m6A modification sites using SRAMP database. We found that TUG1 have more potential m6A modification sites than JPX (Fig. 4B), and TUG1 were verified in the laryngeal cancer cell line and a precancerous cell line. TUG1 showed high expression in laryngeal cancer cell line compared with precancerous cell line. The relation of TUG1 and IGF2BP2 was verified using siRNA to inhibit the laryngeal cancer expression of IGF2BP2. Results from RT-qPCR indicated that both of the two IGF2BP2 siRNAs in TU686 showed a good silenced efficiency and knockdown of TUG1 downregulated the expression level of IGF2BP2 (Fig. 4C).
Discussion
A total of 1171 m6A-related lncRNAs from the TCGA datasets were included in our study to explore their prognostic potential. Finally, 36 m6A-related lncRNAs were identified to have prognostic value in Lasso-Cox analysis, of which 15 were used to establish an m6A-related lncRNA risk model for predicting the OS of HNSCC patients. Subsequently, HNSCC patients were grouped (high-risk and low-risk) based on the median risk score, and patients in the high-risk group exhibited worse clinical results. As shown by ROC analysis, this model was superior to conventional clinical features in OS prediction for HNSCC. According to univariate and multivariate Cox regression analysis, the m6A-related lncRNA model was an independent risk element of OS. Moreover, the perfect consistency between the observed and predicted rates of 1-, 3-, and 5-year OS was shown using a nomogram, which displayed excellent consistency. The risk model that was independently associated with OS was fairly accurate, and this prediction model could identify novel biomarkers for subsequent studies.
Multiple studies have suggested that abnormal m6A-modified lncRNAs may function as regulators in tumour pathogenesis and maintain the malignancy of some tumours. Hu et al. revealed that the interaction between lncRNA DANCR and IGF2BP2 is tightly implicated in inducing pancreatic cancer cell proliferation and tumourigenesis 10. The lnc942-METTL14-CXCR4/CYP1B1 signaling axis has been identified to promote breast cancer cell proliferation and progression 11. A previous study has pointed out that m6A modification may account for FAM225A overexpression in nasopharyngeal carcinoma by improving the stability of FAM225A transcripts 12. Ban et al. found that LNCAROD upregulation in HNSCC might be mediated by dysregulation of m6A modification, which promotes cancer progression by forming a ternary complex with HSPA1A and YBX1 in HNSCC 13. From all the above, m6A modification of lncRNAs may influence cancer initiation and progression, and lncRNAs may serve as competing endogenous RNAs, targeting m6A regulators and thereby influencing aggressive tumour progression. Taken together, we believe that m6A modification is targeted at lncRNAs, and the interactions and functions of lncRNAs and m6A modification should be given more attention in order to identify potential cancer prognostic markers and therapeutic targets.
The mutation profiles in HNSCC were exploited to investigate whether these 15 m6A-related lncRNAs were immunotherapeutic targets or regulators of TME cell infiltration levels. We found a significant negative correlation between the expression of 15 m6A-related lncRNAs and 28 TME cell infiltration, which was consistent with the results of survival analyses for these key molecules. After testing whether these molecules could mediate TME cell infiltration patterns and resistance to anti-checkpoint immunotherapy, 7 key molecules were found to be positively correlated with the expression of immune checkpoint molecules (CTLA-4 and PD1). Immunotherapy and immune cells are inseparable. Growing evidence has demonstrated that the tumour immune microenvironment, tumour gene mutation, and gene regulation are vital factors in tumour development and prognosis. As shown previously, patients treated with CTLA-4 and PD1 immunotherapeutic agents targeting CD8+ T-cell surface receptors have better prognosis 14.
In the present study, 36 m6A-related prognostic lncRNAs from 497 HNSCC patients were identified, of which 15 were included in the m6A-related lncRNA risk model. Furthermore, 7 m6A-related prognostic lncRNAs were shown to be connected with mutated genes. Meanwhile, the m6A-related lncRNA risk model constructed effectively assesses the survival prognosis of patients with HNSCC, providing physicians with optional biomarkers for evaluating therapeutic efficacy. In addition, the 7 m6A-related lncRNA may become new targets for immunotherapy of HNSCC, and it can facilitate the development of individualised treatment, thereby potentially improving survival rates.
LncRNA TUG1, as an oncogenic lncRNA, is highly expressed in multiple human malignancies, such as hepatocellular carcinoma 15, lung adenocarcinoma 16, and laryngeal cancer 17, and TUG1 not only participates in signaling pathways but also mediates multiple miRNAs to promote cancer progression. For example, TUG1 activates tumourigenesis by targeting the miR-138-5p-HIF1A axis in lung adenocarcinoma 16, targeting the miR-137/AKT2 axis in hepatocellular carcinoma 15 and regulating the YAP pathway in renal cell carcinoma 18. However, how m6A effectors and TUG1 get involved in HNSCC progression remains unclear. In the present study, TUG1 acted as an oncogene and appears to be a prognostic biomarker of HNSCC, which is consistent with the results of previous studies 17. TUG1, as an m6A-related lncRNA, also shows a correlation of somatic mutations in CASP8, FAT1, KMT2D, NSD1, and PIK3CA, which are frequently mutated driver genes with poor survival in HNSCC 19-21. According to the results in our study, it was speculated that m6A modification activated TUG1 and promoted tumour progression.
The lncRNA JPX, also known as LINC00183, is an important lncRNA that inactivates the X chromosome 22. JPX regulates tumour cell proliferation and apoptosis. JPX overexpression leads to poor prognosis in oral squamous cell carcinoma 23 and inhibited apoptosis through activating the PI3K/Akt/mTOR signaling in ovarian cancer cells 24. Consistently, the risk model in this study also found that JPX predicted unfavourable prognosis and was associated with PIK3CA.
As reported previously, lncRNA SAP30L-AS1 is highly expressed in prostate cancer and drives malignancy by repressing SAP30L 25.
Several lncRNAs have been reported to be associated with cancer progression. However, the role of lncRNAs in mutation and lncRNA interaction with m6A-related genes in HNSCC has not been fully elucidated. This study aimed to provide insights into identifying lncRNAs that are specifically modified by m6A and how they work during HNSCC progression.
Nevertheless, our study has some limitations. Firstly, only TCGA datasets were included. Our results were divided and validated, and more independent HNSCC cohorts should be used to validate the identified prognostic m6A-related lncRNAs. Secondly, given the marked heterogeneity of HNSCC, our model may exhibit some discrepancies from actual outcomes in future clinical applications. Therefore, we still require detailed stratified analysis on samples from each anatomical site of HNSCC in subsequent studies. Thirdly, their expressions in paired samples were not confirmed to validate the stability of key m6A-related lncRNAs expression in HNSCC tissues. The roles of lncRNAs and their interactions with m6A genes should be examined. If the above aspects could be improved in the future, the persuasiveness of the above results can be increased.
Conclusions
In summary, this study evidenced that 15 m6A-related lncRNAs were notably associated with prognosis and microenvironment immune cell infiltration in patients with HNSCC. The m6A-related lncRNAs risk model could act as an autonomous prognostic factor for predicting the OS of HNSCC patients. This study also provides new ideas for immunotherapeutic strategies and mechanisms underlying m6A modification of lncRNAs in HNSCC.
Conflict of interest statement
The authors declare no conflict of interest.
Funding
This work was supported by the National Natural Science Foundation of China (No. 81870711 and No. 82002895).
Author contributions
WY: conceptualization, resources, formal analysis, writing - original draft; QT: verification, writing - review & editing, funding acquisition; YQ: verification, formal analysis, writing - review & editing; ML: resources, supervision; XT: conceptualization, methodology, investigation, formal analysis, writing - review & editing, supervision; TY: conceptualization, resources, writing - review & editing, supervision.
Ethical consideration
This study was approved by the Institutional Ethics Committee of The Second Xiangya Hospital of Central South University, and the approval number was 2019-R008.
The research was conducted ethically, with all study procedures being performed in accordance with the requirements of the World Medical Association’s Declaration of Helsinki.
Written informed consent was obtained from each participant for study participation and data publication.
History
Received: March 25, 2024
Accepted: October 9, 2024
Figures and tables
Figure 1. The findings of testing and entire sets. The distribution of risk grades, pattern of survival status and survival time, and expression of the 15 m6A-related lncRNAs in testing sets (A) and entire sets (B). Kaplan-Meier survival curves of the OS of patients in the testing set (C) and entire set (D); (E) Stratification of Kaplan-Meier curves in the TCGA entire set; (F) Kaplan-Meier curves of OS differences stratified by 15 m6A-related lncRNAs.
Figure 2. Mutation profile landscape in HNSCC samples. (A) Waterfall plot; (B) Various types of mutation on the basis of various categories; SNV class of mutation; Mutation per sample; Variant classification summary; different colours denote different mutations and top ten mutated genes; (C) The correlations between top 30 mutated genes and the 15 m6A-related lncRNAs; (D) Sankey relational diagram demonstrating the relation among mutated genes, prognostic m6A-related lncRNAs and m6A genes.
Figure 3. (A) Differences in 28 TME infiltration cells between normal and HNSCC tissues (*p < 0.05; **p < 0.01; ***p < 0.001). (B) The correlation between 15 prognostic m6A-related lncRNAs and every TME infiltration cells; (C) The six key molecules had positive correlation with CTLA4 and PD-1; (D) The correlation between RP11_588K22.2 expression and expression of CTLA4 and PD-L2; (E-F) Univariate and multivariate analyses of the clinical characteristics and risk score with the OS; (G) Nomogram based on clinical characteristics and risk score; (H) ROC curves for predicting 1-, 3-, and 5-year OS.
Figure 4. (A) Expression profile of TUG1, JPX and IGF2BP2 between normal and HNSCC samples in the TCGA dataset (top) and four laryngeal cancer tissue samples (bottom); (B) Prediction score of m6A distribution according to the sequence-based RNA adenosine methylation site predictor (SRAMP) algorithm. Y axis presents the combined score at different levels of high, moderate, and low probability. Vertical bars show the score for predictive m6A sites; (C) TUG1 expression between human bronchial epithelial cell line 16HBE and laryngeal cancer cell line TU686 (left). TU686 cells were transfected with siRNAs targeting IGF2BP2 (si IGF2BP2 #1 and si IGF2BP2 #2) or normal control siRNAs (siCtrl) and the expression of IGF2BP2 (middle) and TUG1(right) were examined by RT-qPCR. *p < 0.05; **p < 0.01; ***p < 0.001.
References
- Sun S, Wu Y, Guo W. STAT3/HOTAIR signaling axis regulates HNSCC growth in an EZH2-dependent manner. Clin Canc Res. 2018;24:2665-2677. doi:https://doi.org/10.1158/1078-0432.Ccr-16-2248
- Lu S, Sun Z, Tang L. LINC00355 promotes tumor progression in HNSCC by hindering microRNA-195-mediated suppression of HOXA10 expression. Mol Ther Nucl Acids. 2020;19:61-71. doi:https://doi.org/10.1016/j.omtn.2019.11.002
- Shi H, Wei J, He C. Where, when, and how: context-dependent functions of RNA methylation writers, readers, and erasers. Mol Cell. 2019;74:640-650. doi:https://doi.org/10.1016/j.molcel.2019.04.025
- Haruehanroengra P, Zheng Y, Zhou Y. RNA modifications and cancer. RNA Biol. 2020;17:1560-1575. doi:https://doi.org/10.1080/15476286.2020.1722449
- Hsu P, Shi H, He C. Epitranscriptomic influences on development and disease. Genome Biol. 2017;18. doi:https://doi.org/10.1186/s13059-017-1336-6
- Lan Y, Liu B, Guo H. The role of M(6)A modification in the regulation of tumor-related lncRNAs. Mol Ther Nucl Acids. 2021;24:768-779. doi:https://doi.org/10.1016/j.omtn.2021.04.002
- Zhang H, Zhao L, Li S. N6-Methylandenosine-related lncRNAs in tumor microenvironment are potential prognostic biomarkers in colon cancer. Front Oncol. 2021;11. doi:https://doi.org/10.3389/fonc.2021.697949
- Malla R, Padmaraju V, Marni R. Natural products: potential targets of TME related long non-coding RNAs in lung cancer. Phytomedicine. 2021;93. doi:https://doi.org/10.1016/j.phymed.2021.153782
- Cao R, Yuan L, Ma B. An EMT-related gene signature for the prognosis of human bladder cancer. J Cell Mol Med. 2020;24:605-617. doi:https://doi.org/10.1111/jcmm.14767
- Hu X, Peng W, Zhou H. IGF2BP2 regulates DANCR by serving as an N6-methyladenosine reader. Cell Death Differ. 2020;27:1782-1794. doi:https://doi.org/10.1038/s41418-019-0461-z
- Sun T, Wu Z, Wang X. LNC942 promoting METTL14-mediated m(6)A methylation in breast cancer cell proliferation and progression. Oncogene. 2020;39:5358-5372. doi:https://doi.org/10.1038/s41388-020-1338-9
- Zheng Z, Li Z, Zhou G. Long noncoding RNA FAM225A promotes nasopharyngeal carcinoma tumorigenesis and metastasis by acting as ceRNA to sponge miR-590-3p/miR-1275 and upregulate ITGB3. Canc Res. 2019;79:4612-4626. doi:https://doi.org/10.1158/0008-5472.Can-19-0799
- Ban Y, Tan P, Cai J. LNCAROD is stabilized by m6A methylation and promotes cancer progression via forming a ternary complex with HSPA1A and YBX1 in head and neck squamous cell carcinoma. Mol Oncol. 2020;14:1282-1296. doi:https://doi.org/10.1002/1878-0261.12676
- Luke J, Flaherty K, Ribas A. Targeted agents and immunotherapies: optimizing outcomes in melanoma. Nat Rev Clin Oncol. 2017;14:463-482. doi:https://doi.org/10.1038/nrclinonc.2017.43
- Zhao W, Jiang X, Yang S. lncRNA TUG1 promotes cell proliferation, migration, and invasion in hepatocellular carcinoma via regulating miR-29c-3p/COL1A1 axis. Cancer Manag Res. 2020;12:6837-6847. doi:https://doi.org/10.2147/cmar.S25662416.
- Li K, Niu H, Wang Y. LncRNA TUG1 contributes to the tumorigenesis of lung adenocarcinoma by regulating miR-138-5p-HIF1A axis. Int J Immunopathol Pharmacol. 2021;35. doi:https://doi.org/10.1177/20587384211048265
- Zhuang S, Liu F, Wu P. Upregulation of long noncoding RNA TUG1 contributes to the development of laryngocarcinoma by targeting miR-145-5p/ROCK1 axis. J Cell Biochem. 2019;120:13392-13402. doi:https://doi.org/10.1002/jcb.28614
- Liu S, Yang Y, Wang W. Long noncoding RNA TUG1 promotes cell proliferation and migration of renal cell carcinoma via regulation of YAP. J Cell Biochem. 2018;119:9694-9706. doi:https://doi.org/10.1002/jcb.2728419.
- Zwirner K, Hilke F, Demidov G. Radiogenomics in head and neck cancer: correlation of radiomic heterogeneity and somatic mutations in TP53, FAT1 and KMT2D. Strahlenther Onkol. 2019;195:771-779. doi:https://doi.org/10.1007/s00066-019-01478-x
- Uzunparmak B, Gao M, Lindemann A. Caspase-8 loss radiosensitizes head and neck squamous cell carcinoma to SMAC mimetic-induced necroptosis. JCI Insight. 2020;5. doi:https://doi.org/10.1172/jci.insight.139837
- Farhangdoost N, Horth C, Hu B. Chromatin dysregulation associated with NSD1 mutation in head and neck squamous cell carcinoma. Cell Rep. 2021;34. doi:https://doi.org/10.1016/j.celrep.2021.108769
- Tian D, Sun S, Lee J. The long noncoding RNA, Jpx, is a molecular switch for X chromosome inactivation. Cell. 2010;143:390-403. doi:https://doi.org/10.1016/j.cell.2010.09.049
- Yao Y, Chen S, Lu N. LncRNA JPX overexpressed in oral squamous cell carcinoma drives malignancy via miR-944/CDH2 axis. Oral Dis. 2021;27:924-933. doi:https://doi.org/10.1111/odi.13626
- Li J, Feng L, Tian C. Long noncoding RNA-JPX predicts the poor prognosis of ovarian cancer patients and promotes tumor cell proliferation, invasion and migration by the PI3K/Akt/mTOR signaling pathway. Eur Rev Med Pharmacol Sci. 2018;22:8135-8144. doi:https://doi.org/10.26355/eurrev_201812_16505
- Qin X, Zhu W, Lu A. Long non-coding RNA SAP30L-AS1 promotes prostate cancer growth through repressing SAP30L. Gene. 2019;690:120-128. doi:https://doi.org/10.1016/j.gene.2018.12.047
Downloads
License
This work is licensed under a Creative Commons Attribution-NonCommercial-NoDerivatives 4.0 International License.
Copyright
Copyright (c) 2024 Società Italiana di Otorinolaringoiatria e chirurgia cervico facciale
How to Cite
- Abstract viewed - 483 times
- PDF downloaded - 239 times