Thyroid
Vol. 44: Issue 4 - August 2024
Predicting excellent response to radioiodine in differentiated thyroid cancer using machine learning
Abstract
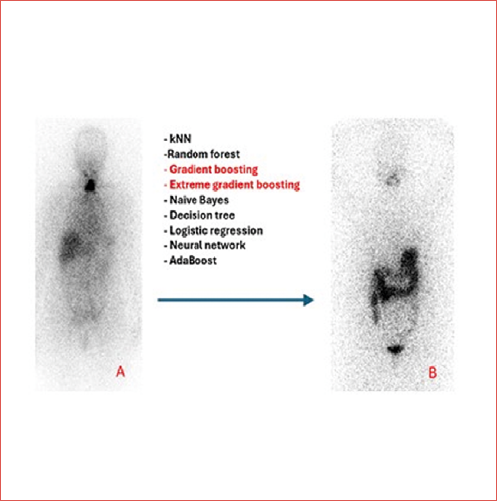
Objective. If excellent response (ER) occurs after radioactive iodine (RAI) treatment in patients with differentiated thyroid carcinoma (DTC), the recurrence rate is low. Our study aims to predict ER at 6-24 months after RAI by using machine learning (ML) methods in which clinicopathological parameters are included in patients with DTC without distant metastasis.
Methods. Treatment response of 151 patients with DTC without distant metastasis and who received RAI treatment was determined (ER/nonER). Thyroidectomy ± neck dissection pathology data, laboratory, and imaging findings before and after RAI treatment were introduced to ML models.
Results. After RAI treatment, 118 patients had ER and 33 had nonER. Before RAI treatment, TgAb was positive in 29% of patients with ER and 55% of patients with nonER (p = 0.007). Eight of the ML models predicted ER with high area under the ROC curve (AUC) values (> 0.700). The model with the highest AUC value was extreme gradient boosting (AUC = 0.871), the highest accuracy shown by gradient boosting (81%).
Conclusions. ML models may be used to predict ER in patients with DTC without distant metastasis.
Introduction
Differentiated thyroid cancer (DTC) is the most common malignant endocrine neoplasm. The incidence of DTC has increased due to the increased frequency of use of ultrasonography 1. The most crucial component of the treatment of DTC is total or near-total thyroidectomy. According to preoperative evaluation, central and/or lateral neck dissection can be performed. Ablation/adjuvant therapy/metastasis treatment can be performed using radioactive iodine (RAI) according to the disease stage and risk assessment after surgery 2.
The American Thyroid Association (ATA) recommends evaluating treatment response after RAI under four headings: 1) excellent response (ER), 2) biochemical incomplete response (BIR), 3) structural incomplete response (SIR), and 4) indeterminate response (IDR). The recurrence rate in patients with ER at one end of the treatment response scale is 1-4%; distant metastases can be seen in 50% of patients with SIR at the other end. Structural disease may also occur in patients with BIR and IDR 2.
During follow-up, patients with ER and physicians following these patients are more comfortable psychologically; this is the opposite for BIR, SIR, and IDR. Therefore, it is crucial to predict ER after RAI. There are some established clinicopathological predictors for ER 3-5, ablation success 6,7, and treatment failure 8-11 after RAI treatment. Our study aims to predict ER 6-24 months after RAI by using machine learning (ML) methods in which clinicopathological parameters are included in patients with DTC without distant metastasis.
Materials and methods
Patients
All patients who received RAI treatment in our department between 2019 and 2022 due to DTC were identified (436 patients). The following patients were excluded: 1) patients with distant metastasis, 2) patients with missing thyroglobulin (Tg), antithyroglobulin antibody (TgAb), neck ultrasonography (USG) data in the hospital registry system during the follow-up period, 3) patients exposed to iodinated contrast material in the three months before RAI. Finally, 151 patients were included in the study.
Radioactive iodine treatment protocol
Levothyroxine was discontinued for four weeks in patients who started using levothyroxine after total/near total thyroidectomy ± central/lateral neck dissection, while some patients used triiodothyronine in the first two weeks. TSH measured on the day of RAI treatment was > 30 IU/mL in all patients. An iodine-restricted diet was applied for two weeks before RAI treatment. RAI doses were determined according to the recurrence risk of the patients (30-200 mCi). Whole body scintigraphy (WBS) images were obtained using Mediso AnyScan 2013-2016 dual-head gamma cameras on days 5-9 after RAI (with high-energy collimators, peak 364 keV; window 15%).
Evaluation of treatment response
In our clinic, non-stimulated thyroglobulin (nsTg) measurements are used in the routine follow-up of patients with DTC after RAI to avoid hypothyroidism caused by levothyroxine withdrawal and due to the high cost of recombinant human TSH (rhTSH). Patients were divided into two groups (ER and nonER) according to the treatment responses obtained after RAI treatment. For ER, it was necessary to have the following criteria, also specified in the ATA 2015 guideline: negative imaging (WBS and neck USG) and nsTg < 0.2 ng/mL in the absence of TgAb. According to the ATA 2015 guidelines, patients with BIR, SIR, or IDR were classified as nonER 2.
Laboratory measurements
An automated immunoassay (Centaur Systems, Siemens) method was used to measure TSH and TgAb, and the enzyme immunoassay method was used to measure Tg.
Statistical analysis
IBM SPSS 27 (IBM Corp. Released 2020. IBM SPSS Statistics for Macintosh, Version 27.0. Armonk, NY: IBM Corp) was used for statistical analysis.
The conformity of the variables to normal distribution was evaluated using the Kolmogorov-Smirnov test. Categorical variables were expressed as numbers and percentages. Continuous variables were expressed as the mean ± standard deviation (SD) or the median and minimum-maximum. The effect of continuous variables on ER was analysed using the “independent groups t-test” or the “Mann-Whitney U” test. The effect of categorical variables on ER was analysed using the chi-square test.
Continuous variables in our study were patient age, tumour size, and RAI dose. The categorical variables in our study were: gender, presence of aggressive histopathological subtype, multifocality, lymphatic invasion, vascular invasion, perineural invasion, positive surgical margin, presence of histopathologically proven cervical lymph node metastasis, ATA risk group (low, moderate or high), stimulated thyroglobulin (sTg) before RAI (< 10 ng/mL or ≥ 10 ng/mL) and TgAb (positive or negative), time between total thyroidectomy and RAI treatment (titRAI) (< 3 months or ≥ 3 months), and presence of thyroid tissue on WBS after RAI treatment.
Machine learning
The ability of ML methods to predict ER was evaluated with the Orange data mining toolbox (Version 3.34.0). The Synthetic Minority Over-Sampling TEchnique (SMOTE), one of the oversampling methods, was used to eliminate the imbalance between the number of patients with nonER (n = 34) and ER (n = 119) 12.
Eighty percent of the patients were randomised to the training group and 20% to the testing group. Ten-fold cross-validation was used in the training group. In the preprocessing stage, the data were normalised between 0 and 1. The 10 variables that best predicted ER were determined by the ANOVA method. Using the mentioned 10 variables, the predictive power of k-nearest neighbors (kNN), random forest, gradient boosting, extreme gradient boosting, naive Bayes, decision tree, logistic regression, neural network, and AdaBoost methods for ER was investigated on the training group. The knowledge that ML models learned to predict ER in the training group was validated in the testing group. The workflow of the design of our study is summarised in Figure 1.
Results
In all, 110 of the patients were females and 41 were males. All patients had papillary carcinoma. While 118 patients had ER after RAI treatment, 33 had nonER. Detailed information about the patient population is shown in Table I.
TgAb was positive in 55% of patients with ER and 29% with nonER before RAI treatment (p = 0.007). Other continuous or categorical variables did not significantly differ between patients with and without ER (Tab. I).
ML methods learned the relationships between the training group data and the ER status and used the learned relationships on the testing group data (Tab. II). Tree, random forest, neural network, naive Bayes, logistic regression, gradient boosting, extreme gradient boosting, and AdaBoost were able to identify patients with ER with high AUC values (> 0.700) (Tab. III). The model with the highest AUC value was extreme gradient boosting (AUC = 0.871). The model that determined the ER as having the highest accuracy (81%) was gradient boosting (Cover figure).
Discussion
If ER is obtained after initial treatment (total or near-total thyroidectomy ± RAI) in DTC, the recurrence rate is 1-4%.2 There are studies in the literature aiming to predict the success or failure of RAI treatment using classical statistical methods. In this study, we aimed to predict ER using different ML methods after RAI treatment.
Barres et al. followed 1093 patients with DTC for a median of 5 years. Predictors of ER were found to be sTg < 1 μg/L before RAI treatment and more than a 60% decrease in Tg after RAI treatment 3. The same study determined a correlation between sTg and disease persistence, locoregional recurrence, and distant metastasis.
Giovanella et al. ablated 193 low-risk DTC patients using 1.1 GBq (30 mCi) RAI. They found that the rate of no residue in Tc-99m pertechnetate thyroid scintigraphy was higher in patients with successful ablation (52-96%, p < 0.001). They also determined that sTg values before RAI treatment were lower in patients with successful ablation (2.7-5.8 ng/mL, p < 0.010) 6.
In the study by Yun et al. on 1228 patients with DTC, nonER was more frequent after RAI treatment if there were more than 5 metastatic lymph nodes. In addition, if the metastatic lymph node ratio (metastatic lymph node number/number of dissected lymph nodes) was greater than 0.3, nonER was more frequent after RAI treatment 13.
Park et al. tried to predict failure of RAI therapy in 132 patients with DTC. Although univariate analysis showed T category, tumour size, and the preablation sTg to be associated with therapeutic failure, only preablation sTg was identified as an independent risk factor indicating treatment failure in the multivariate analysis 8.
Prpic et al. investigated the causes of RAI treatment failure in 704 DTC patients.14 Treatment failure was more common in younger patients (< 53 years), patients with preablation sTg > 2.4 ng/mL, patients with N1a, and those with extracapsular invasion of the metastatic lymph nodes.
Dong et al. ablated 506 patients with low/intermediate risk DTC with 1.1 GBq (30 mCi) or 3.7 GBq (100 mCi). They reported that preablation sTG ≥ 10 ng/mL is a risk factor for incomplete response. They concluded that RAI dose is not a risk factor for incomplete response in low/intermediate risk patients, and that 1.1 GBq RAI dose can be used for ablation.9
Iizuka et al. showed that preablation sTg > 4 ng/mL was associated with ablation failure in their study of 119 intermediate/high-risk DTC patients. In the same study, 1100 MBq (30 mCi) RAI was used in patients with intermediate risk, and 2960-3700 MBq (80-100 mCi) RAI was used in high-risk patients for ablation. The authors found that different RAI doses did not affect ablation success 15.
In this study, as a result of classical statistical analysis with 151 patients, only the positivity rate of TgAb differed between patients with ER and nonER after RAI treatment. Preablation sTg, which predicts treatment failure with different threshold values in many studies, did not differ between ER and nonER patients in our study.
The effect of the time between RAI and thyroidectomy (titRAI) on treatment success is unclear. While some studies showed that treatment success decreased when titRAI was more prolonged, some studies reported that titRAI did not affect treatment success 16-21. In our study, no difference was found in ER rates in patients with titRAI < 3 months and ≥ 3 months.
Considering the power of ML models to predict ER, which is the primary purpose of this study (Tab. III), it is seen that models other than kNN are successful. Extreme gradient boosting had the highest AUC value (0.871), while gradient boosting had the highest accuracy (81%). When basic statistical methods were used, we found that the variables other than TgAb positivity did not differ significantly between patients with ER and nonER. However, ER could be accurately predicted when these variables were transferred to ML models. ML models can produce impressive results from data that seems unimportant/less important by establishing complex relationships between independent variables. Some researchers have shown that ML in different cancer types produces successful results in diagnosis, treatment response evaluation, and determining prognosis 22-25. ML is not currently an accepted method for prognostication in the clinical management of DTC, but it can generate useful prognostic information for both clinicians and patients. If clinicians can obtain prognostic information through ML in DTC, they can be more helpful to patients. For example, higher RAI doses may be preferred in patients with poor prognosis, and clinical follow-up may be performed at closer intervals. The opposite of this situation is also true.
There are some limitations of our study. Due to the retrospective design and the small number of patients, the number of patients with ER and nonER could not be randomised in a balanced way. For this reason, SMOTE, one of the oversampling methods, was used to ensure the dataset was balanced. While evaluating the response of RAI treatment, we did not use sTg and RAI WBS; instead, we preferred nsTg and neck USG for these reasons: RAI WBS is not a routinely recommended method to assess treatment response under current knowledge 2. The use of rhTSH to measure sTg increases healthcare costs and measurement of sTg with levothyroxine discontinuation causes symptoms of hypothyroidism in patients. Lastly, measurement of the nsTg is a reliable method to evaluate treatment response 26-28. In our study, we aimed to predict excellent response using ML in the early period (6-24 months) after RAI. However, recurrence in DTC usually occurs after long-term follow-up. Some of the patients in our study did not have thyroid scintigraphy to determine residual thyroid tissue before RAI, so the effect of the amount of residual thyroid tissue on ER/nonER status could not be evaluated.
Conclusions
Although so far not an accepted prognostic tool, ML methods can successfully predict ER after RAI treatment in DTC 29. In patients with TgAb positivity before RAI treatment, nonER may be seen more frequently. The results of our study should be supported by multicentre studies with larger numbers of patients.
Conflict of interest statement
The authors declare no conflict of interest.
Funding
This research did not receive any specific grant from any funding agency in the public, commercial or not-for-profit sector.
Author contributions
OB: concept, design, literature search, data acquisition, data analysis, statistical analysis, manuscript preparation, manuscript editing, and manuscript review; DN: concept, design, data acquisition, manuscript editing, and manuscript review.
Ethical consideration
This study was approved by the Ethics Committee of Recep Tayyip Erdoğan University Faculty of Medicine and conducted according to the principles of the Declaration of Helsinki (Decision date: 01.06.2023, approval number: 2023/141). All patients gave informed consent before RAI treatment, participation and data publication.
History
Received: March 28, 2024
Accepted: May 10, 2024
Figures and tables
Figure 1. Workflow of patient selection and machine learning process. RAI: radioactive iodine; ER: excellent response.
Variable (mean ± SD) | Patients with ER | Patients with nonER | p value |
---|---|---|---|
Age | 46 ± 12 | 45 ± 12 | 0.547 |
Largest diameter of tumour (mm) | 19.8 ± 12.4 | 16.9 ± 10.7 | 0.197 |
Preablation sTg (ng/mL) | 5.3 ± 10.2 | 5.8 ± 12.8 | 0.090 |
Radioactive iodine dose (mCi) | 97 ± 32 | 110 ± 37 | 0.058 |
Variable N (%) | Patients with ER | Patients with nonER | p value |
Sex | 0.178 | ||
Female | 89(75) | 21(64) | |
Male | 29(25) | 12(26) | |
Histology | 1.000 | ||
Papillary | 118(100) | 33(100) | |
Other | - | - | |
Subtype with poor prognosis * | 0.587 | ||
Yes | 37(31) | 12(36) | |
No | 81(69) | 21(64) | |
Multifocality | 0.986 | ||
Yes | 86(73) | 24(73) | |
No | 32(27) | 9(27) | |
Positive surgical margin | 0.226 | ||
Yes | 24(20) | 10(30) | |
No | 94(80) | 23(70) | |
Lymphatic invasion | 0.146 | ||
Yes | 28(24) | 12(36) | |
No | 90(76) | 21(64) | |
Vascular invasion | 0.547 | ||
Yes | 11(9) | 2(6) | |
No | 107(91) | 31(94) | |
Perineural invasion | 0.421 | ||
Yes | 8(7) | 1(3) | |
No | 110(93) | 32(97) | |
Extrathyroidal extension | 0.700 | ||
Yes | 4(3) | 1(3) | |
No | 114(97) | 32(97) | |
ATA 2015 risk group | 0.119 | ||
Low | 61(52) | 11(23) | |
Intermediate | 53(45) | 21(64) | |
High | 4(3) | 1(3) | |
T category | 0.620 | ||
T1-T2 | 112(95) | 32(97) | |
T3-T4 | 6(5) | 1(3) | |
N category | 0.138 | ||
N0 | 102(86) | 25(76) | |
N1a/N1b | 16(14) | 8(24) | |
TgAb | 0.007 | ||
Positive | 34(29) | 18(55) | |
Negative | 82(71) | 15(45) | |
titRAİ | 0.223 | ||
< 3 months | 87(74) | 21(64) | |
≥ 3 months | 31(26) | 12(36) | |
Remnant tissue on postablation WBS | 0.783 | ||
Yes | 109(92) | 30(91) | |
No | 9(8) | 3(9) | |
* Like tall cell, hobnail variant, columnar cell carcinoma. | |||
ER: excellent response after radioactive iodine, nonER: treatment response patterns other than excellent response, SD: standard deviation, sTg: stimulated thyroglobulin, ATA: American Thyroid Association, TgAb: thyroglobulin antibody, titRAI: time interval between radioactive iodine and thyroidectomy, WBS: whole body scintigraphy. |
Model | AUC | Sensitivity (%) | Specificity (%) | Accuracy (%) |
---|---|---|---|---|
kNN | 0.731 | 68 | 68 | 68 |
Tree | 0.734 | 71 | 66 | 68 |
Random forest | 0.809 | 79 | 76 | 77 |
Neural network | 0.801 | 75 | 73 | 74 |
Naive bayes | 0.694 | 80 | 49 | 65 |
Logistic regression | 0.748 | 77 | 65 | 71 |
Extreme gradient boosting | 0.763 | 74 | 72 | 73 |
Gradient boosting | 0.799 | 74 | 74 | 74 |
AdaBoost | 0.757 | 74 | 67 | 70 |
ER: excellent response after radioactive iodine, AUC: area under the curve, kNN: k-nearest neighbors. |
Model | AUC | Sensitivity (%) | Specificity (%) | Accuracy (%) |
---|---|---|---|---|
kNN | 0.670 | 48 | 83 | 66 |
Tree | 0.755 | 65 | 83 | 74 |
Random forest | 0.857 | 57 | 83 | 70 |
Neural network | 0.864 | 52 | 71 | 62 |
Naive bayes | 0.777 | 87 | 58 | 72 |
Logistic regression | 0.713 | 78 | 58 | 68 |
Extreme gradient boosting | 0.865 | 70 | 83 | 77 |
Gradient boosting | 0.850 | 83 | 79 | 81 |
AdaBoost | 0.764 | 65 | 88 | 77 |
ER: excellent response after radioactive iodine, AUC: area under the curve, kNN: k-nearest neighbors. |
References
- Davies L, Welch H. Increasing incidence of thyroid cancer in the United States, 1973-2002. JAMA. 2006;295:2164-2167. doi:https://doi.org/10.1001/jama.295.18.2164
- Haugen B, Alexander E, Bible K. 2015 American Thyroid Association Management Guidelines for adult patients with thyroid nodules and differentiated thyroid cancer: the American Thyroid Association Guidelines Task Force on thyroid nodules and differentiated thyroid cancer. Thyroid. 2016;26:1-133. doi:https://doi.org/10.1089/thy.2015.0020
- Barres B, Kelly A, Kwiatkowski F. Stimulated thyroglobulin and thyroglobulin reduction index predict excellent response in differentiated thyroid cancers. J Clin Endocrinol Metab. 2019;104:3462-3472. doi:https://doi.org/10.1210/jc.2018-02680
- Lan W, Gege Z, Ningning L. Negative remnant 99m Tc-pertechnetate uptake predicts excellent response to radioactive iodine therapy in low- to intermediate-risk differentiated thyroid cancer patients who have undergone total thyroidectomy. Ann Nucl Med. 2019;33:112-118. doi:https://doi.org/10.1007/s12149-018-1314-4
- Park H, Min J, Bom H. Early stimulated thyroglobulin for response prediction after recombinant human thyrotropin-aided radioiodine therapy. Ann Nucl Med. 2017;31:616-622. doi:https://doi.org/10.1007/s12149-017-1190-3
- Giovanella L, Paone G, Ruberto T. 99m Tc-pertechnetate scintigraphy predicts successful postoperative ablation in differentiated thyroid carcinoma patients treated with low radioiodine activities. Endocrinol Metab. 2019;34:63-69. doi:https://doi.org/10.3803/EnM.2019.34.1.63
- Kim E, Kim T, Kim W. Effects of different doses of radioactive iodine for remnant ablation on successful ablation and on long-term recurrences in patients with differentiated thyroid carcinoma. Nucl Med Commun. 2011;32:954-959. doi:https://doi.org/10.1097/MNM.0b013e32834956ec
- Park H, Jeong G, Kwon S. Stimulated serum thyroglobulin level at the time of first dose of radioactive iodine therapy is the most predictive factor for therapeutic failure in patients with papillary thyroid carcinoma. Nucl Med Mol Imaging. 2014;48:255-261. doi:https://doi.org/10.1007/s13139-014-0282-4
- Dong P, Qu Y, Yang L. Outcomes after radioiodine ablation in patients with thyroid cancer: long-term follow-up of a Chinese randomized clinical trial. Clin Endocrinol (Oxf). 2021;95:782-789. doi:https://doi.org/10.1111/cen.14563
- Liu Y, Li H, Liu J. Unfavorable responses to radioiodine therapy in N1b papillary thyroid cancer: a propensity score matching study. Endocr Pract. 2019;25:1286-1294. doi:https://doi.org/10.4158/EP-2019-0155
- Jeong E, Yoon J, Lee S. Risk factors for indeterminate response after radioactive iodine therapy in patients with differentiated thyroid cancer. Clin Nucl Med. 2019;44:714-718. doi:https://doi.org/10.1097/RLU.0000000000002653
- Chawla N, Bowyer K, Hall L. SMOTE: Synthetic Minority Over-sampling TEchnique. J Artif Intell Res. 2002;16:321-357. doi:https://doi.org/10.1613/jair.953
- Yun C, Xiao J, Cao J. Lymph node metastases > 5 and metastatic lymph node ratio > 0.30 of differentiated thyroid cancer predict response to radioactive iodine. Cancer Med. 2021;10:7610-7619. doi:https://doi.org/10.1002/cam4.4288
- Prpic M, Kust D, Kruljac I. Prediction of radioactive iodine remnant ablation failure in patients with differentiated thyroid cancer: a cohort study of 740 patients. Head Neck. 2017;39:109-115. doi:https://doi.org/10.1002/hed.24550
- Iizuka Y, Katagiri T, Ogura K. Comparison between the different doses of radioactive iodine ablation prescribed in patients with intermediate-to-high-risk differentiated thyroid cancer. Ann Nucl Med. 2019;33:495-501. doi:https://doi.org/10.1007/s12149-019-01357-6
- Higashi T, Nishii R, Yamada S. Delayed initial radioactive iodine therapy resulted in poor survival in patients with metastatic differentiated thyroid carcinoma: a retrospective statistical analysis of 198 cases. J Nucl Med. 2011;52:683-689. doi:https://doi.org/10.2967/jnumed.110.081059
- Tsirona S, Vlassopoulou V, Tzanela M. Impact of early vs late postoperative radioiodine remnant ablation on final outcome in patients with low-risk well-differentiated thyroid cancer. Clin Endocrinol (Oxf). 2014;80:459-463. doi:https://doi.org/10.1111/cen.12301
- Scheffel R, Zanella A, Dora J. Timing of radioactive iodine administration does not influence outcomes in patients with differentiated thyroid carcinoma. Thyroid. 2016;26:1623-1629. doi:https://doi.org/10.1089/thy.2016.0038
- Suman P, Wang C, Abadin S. Timing of radioactive iodine therapy does not impact overall survival in high-risk papillary thyroid carcinoma. Endocr Pract. 2016;22:822-831. doi:https://doi.org/10.4158/EP151088.OR
- Suman P, Wang C, Moo-Young T. Timing of adjuvant radioactive iodine therapy does not affect overall survival in low- and intermediate-risk papillary thyroid carcinoma. Am Surg. 2016;82:807-814. doi:https://doi.org/10.1177/000313481608200
- Li H, Zhang Y, Wang C. Delayed initial radioiodine therapy related to incomplete response in low- to intermediate-risk differentiated thyroid cancer. Clin Endocrinol (Oxf). 2018;88:601-606. doi:https://doi.org/10.1111/cen.13551
- Swanson K, Wu E, Zhang A. From patterns to patients: advances in clinical machine learning for cancer diagnosis, prognosis, and treatment. Cell. 2023;186:1772-1791. doi:https://doi.org/10.1016/j.cell.2023.01.035
- Montenegro C, Paderno A, Ravanelli M. Thyroid cartilage infiltration in advanced laryngeal cancer: prognostic implications and predictive modelling. Acta Otorhinolaryngol Ital. 2024;44:176-182. doi:https://doi.org/10.14639/0392-100X-N2739
- Ferrari M, Mattavelli D, Schreiber A. Does reorganization of clinicopathological information improve prognostic stratification and prediction of chemoradiosensitivity in sinonasal carcinomas? A retrospective study on 145 patients. Front Oncol. 2022;3. doi:https://doi.org/10.3389/fonc.2022.799680
- Resteghini C, Trama A, Borgonovi E. Big data in head and neck cancer. Curr Treat Options Oncol. 2018;19. doi:https://doi.org/10.1007/s11864-018-0585-2
- Szujo S, Bajnok L, Bodis B. The prognostic role of postablative non-stimulated thyroglobulin in differentiated thyroid cancer. Cancers (Basel). 2021;13:1-11. doi:https://doi.org/10.3390/cancers13020310
- Moreno I, Hirsch D, Duskin-Bitan H. Response to therapy assessment in intermediate-risk thyroid cancer patients: is thyroglobulin stimulation required?. Thyroid. 2020;30:863-870. doi:https://doi.org/10.1089/thy.2019.0431
- Rosario P, Mourão G, Calsolari M. Low postoperative nonstimulated thyroglobulin as a criterion for the indication of low radioiodine activity in patients with papillary thyroid cancer of intermediate risk ‘with higher risk features’. Clin Endocrinol. 2016;85:453-458. doi:https://doi.org/10.1111/cen.13024
- Mäkitie A, Alabi R, Ng S. Artificial intelligence in head and neck cancer: a systematic review of systematic reviews. Adv Ther. 2023;40:3360-3380. doi:https://doi.org/10.1007/s12325-023-02527-9
Downloads
License
This work is licensed under a Creative Commons Attribution-NonCommercial-NoDerivatives 4.0 International License.
Copyright
Copyright (c) 2024 Società Italiana di Otorinolaringoiatria e chirurgia cervico facciale
How to Cite
- Abstract viewed - 100 times
- PDF downloaded - 28 times